The Art of expecting the unexpected: The Gemini Robotics way
- Tommaso Pardi
- Mar 15
- 4 min read
Welcome to the Age of Robots That Just Get It
Imagine you’re teaching a robot how to clean your kitchen. You show it how to pick up a plate, wipe down the counter, and maybe even toss a snack wrapper into the bin. Great! Now, what if you ask it to do something completely new, like stack those plates Jenga-style? Most traditional robots would freeze like a deer in headlights. But Gemini robotics seems to shrug (metaphorically) and say, “Well, let's give it a go.”
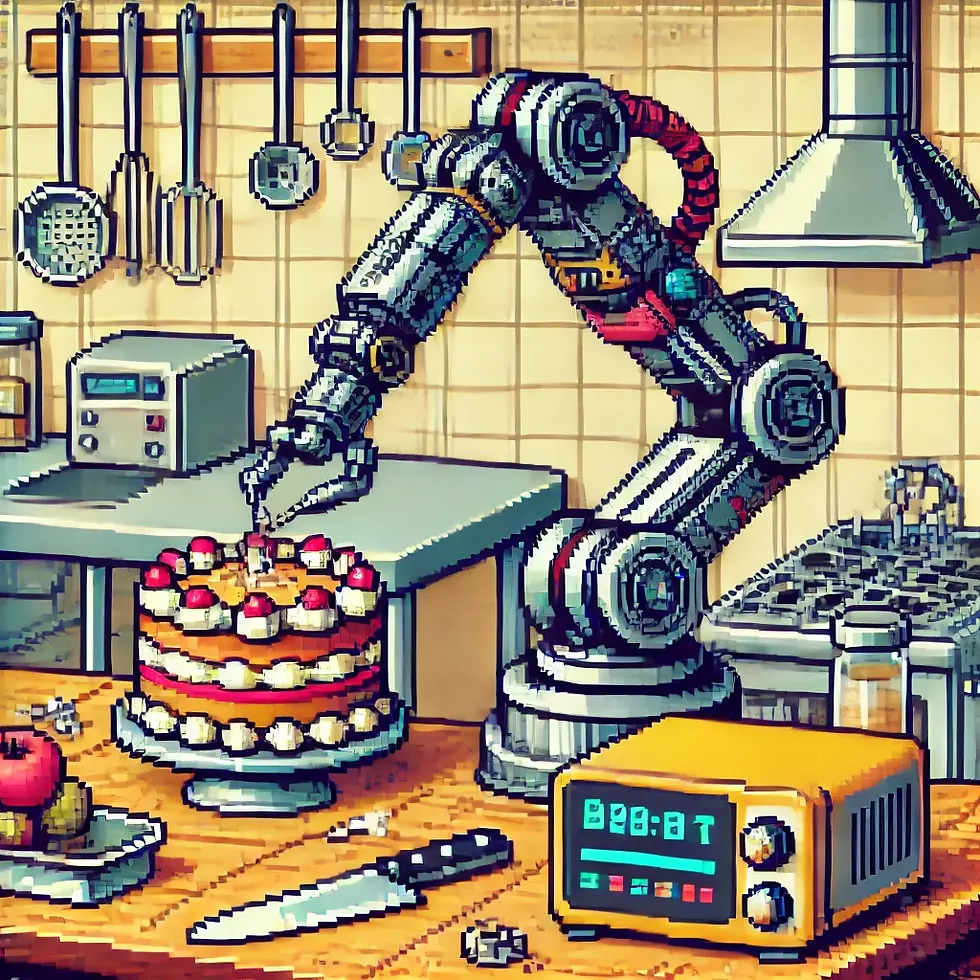
That’s because the last model developed by Google DeepMind, isn’t just another AI-powered machine—it’s a robot system equipped with a world model, meaning it doesn’t just memorise tasks; it understands the world well enough to generalise.
It’s the difference between me memorising a few phrases in Spanish when I go to Spain and actually learning the language. The results? Stunning, especially when it comes to out-of-distribution (OOD) tasks problems it’s never seen before.
Foundational Models and World Models: The Secret Sauce
Foundational models in AI are like all-purpose Swiss Army knives—they're trained on vast amounts of data, making them flexible across many different scenarios. In robotics, this is a game-changer. Instead of programming robots with specific instructions for every possible situation (an if-else nightmare), these models allow for real-time adaptation.
At the core of Gemini Robotics is a powerful world embedding—a deep, structured understanding of the physical world that lets it predict outcomes, plan actions, and think before it moves. This isn’t just theoretical. It’s already happening.
Take Gemini Robotics’ performance on OOD tasks: research suggests it achieves around 75% success on tasks it has never seen before, doubling the performance of existing vision-language-action (VLA) models. Think about that for a second. We’re talking about robots that can adapt to new objects, unfamiliar layouts, and unexpected commands—all without a human holding their metaphorical hand.
Meet the Future: A Robot That Adapts - Gemini Robotics
The magic behind Gemini Robotics is its blend of generality, interactivity, and dexterity:
Generality: Thanks to Gemini 2.0’s deep training, it can pick up on patterns and apply knowledge across different tasks. A robot trained to put a toy in a box can reason that a book should probably go in there too. Simple for us, groundbreaking for AI.
Interactivity: Unlike traditional systems that require strict programming, Gemini Robotics understands conversational language and can adapt dynamically to changes in its environment. Spill your coffee? It’ll adjust its movements rather than knocking everything over.
Dexterity: Gemini Robotics has demonstrated impressive feats like origami folding and packing snacks into Ziploc bags (because clearly, someone at DeepMind really loves meal prep). Its action updates at 50Hz, meaning it can respond 50 times per second—fast enough to be smooth, precise, and eerily human-like in movement.
Why Out-of-Distribution (OOD) Tasks Matter
One of the biggest limitations in robotics has always been generalisation. A robot might perform flawlessly in a carefully controlled lab but completely fails when you change even a small detail in the real world. OOD tasks test a model’s ability to handle these unknowns—can it think on its feet, or does it crumble under pressure?
Gemini Robotics has shown it doesn’t just perform well—it doubles the generalisation capabilities of previous models. That means:
A warehouse robot trained for organising boxes can figure out how to handle irregularly shaped items without panicking.
A home assistant robot can recognise a new brand of dish soap and still understand where to put it.
A manufacturing robot can adjust to slight variations in parts without needing reprogramming.
In short, it moves us one step closer to truly autonomous robots that don’t need endless human micromanagement.
The Unexpected Details: A Robot Ninja?
A fun fact buried in DeepMind’s research is that Gemini Robotics operates at a 50Hz control frequency, meaning it updates its actions 50 times per second. This might not sound impressive until you realise that’s faster than most humans can react. Picture a robot dodging obstacles, grabbing a falling object mid-air, or moving with uncanny fluidity.
Combine that with an end-to-end latency of ~250ms (from perception to action), and we’re looking at one of the most responsive robotics systems ever built. No more jerky, awkward movements—Gemini Robotics moves like it belongs in the real world.
Safety First: The Three Laws (Sort of)
Of course, with great power comes great responsibility. DeepMind is well aware of the risks of unleashing highly autonomous robots, which is why they’ve implemented extensive safety measures:
Collision avoidance to prevent accidents.
Force limitations to ensure human-robot interactions remain safe.
ASIMOV safety benchmarks (yes, named after Isaac Asimov’s Three Laws of Robotics) align robot behaviour with ethical standards.
The end goal? Robots that are helpful, safe, and trustworthy, rather than the nightmare fuel of sci-fi horror movies.
What’s Next?
With partnerships spanning from Agile Robots to Boston Dynamics and Apptronik, Gemini Robotics isn’t just a research project—it’s a real, deployable technology with industry-wide implications. Whether it’s in warehouses, homes, or even medical settings, these robots are poised to revolutionise how AI interacts with the
physical world.
And the best part? We’re only scratching the surface. The ability to handle OOD tasks is the first major leap toward true AI-powered autonomy. Who knows—maybe one day, your household robot will actually understand the mess you call your kitchen, not just blindly follow a script.
ความคิดเห็น